
Image recognition and artificial intelligence in sales and merchandising
We use AI in our products and provide clients with quality based on it. So, let us take a closer look at terms related to artificial intelligence.
It is over 20 years since Garry Kasparov lost to the supercomputer Deep Blue. We can deem that moment to be the beginning of the modern era of artificial intelligence, but when it comes to who is going to write this story, views are divided. We use AI methods in our products and provide our clients with quality based on these solutions. So, let us take a closer look at some basic terms related to artificial intelligence.
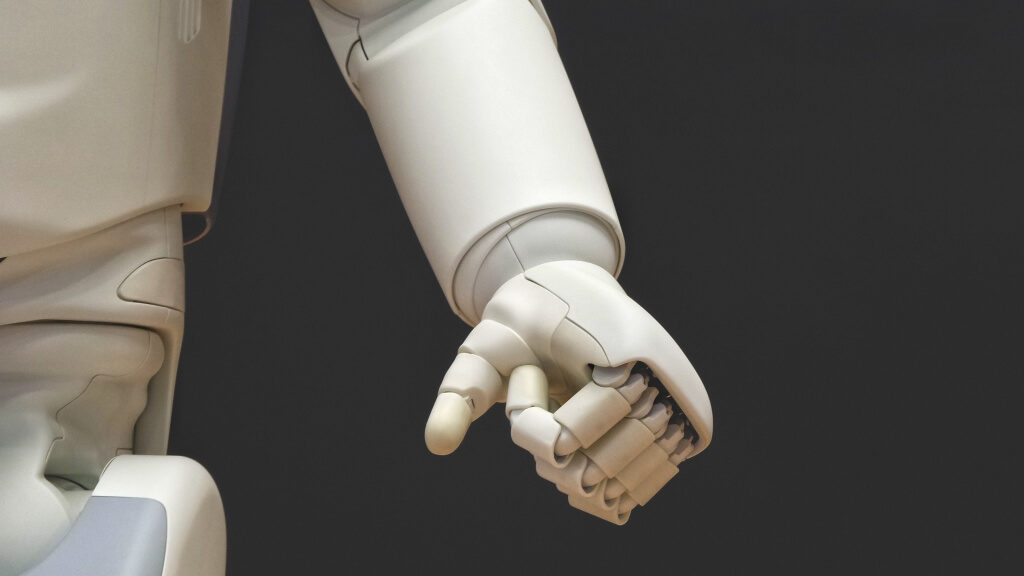
Where to look for artificial intelligence?
Artificial intelligence as a field of science has its origins in the mid twentieth century and it is connected with the names Alan Turing (we recommend the movies The Imitation Games and Ex Machina!) and John McCarthy. In the most simplistic terms, the idea of artificial intelligence is to make human creations behave, learn and solve problems, like humans. Or even better than a human being. Because although you cannot put yourself up by your bootstraps (neither physically, nor intellectually), there are ways to increase the efficiency of devices to the point beyond the boundaries of human imagination physical capability. According to the most ambitious concept of “strong artificial intelligence”, machines could even feel human emotions and display impulsiveness. However, let us come back to here and now.
In order to understand what artificial intelligence really is, we can use the scheme below:

A set of all algorithms for solving problems commonly associated with human intelligence and executed by machines is Artificial Intelligence (AI). Processing data in a fast and repeatable way makes it easier for people to draw conclusions and speeds up problem solving. The first computer chess programs – as well as other computer games – used AI in this sense (you still cannot provoke the computer to knock over the chessboard :). They are algorithms following the principle: if something happens, do this; otherwise do that or do nothing.
Learn more:
- Energy drinks 2024: industry view through the lens of AI
- How to maintain merchandising standards and a perfect store thanks to AI [VIDEO]
- Price on shelf different from price at checkout – what should the shop do?
- How to implement Shelf Recognition tool, organize the processes and win the market?
A level deeper, there is Machine Learning (ML). This method is able to solve problems and answer questions using complex statistical tools. So, on the horizon there appears probabilistic reasoning and other inspiring mind “accelerators” that help to reach solutions obscured by a huge amount of data. Machine learning assumes that data processed earlier has an influence on the analysis of data processed later.

An interesting example of machine learning is the Zillow portal, which supports real estate trade, where prices are estimated using artificial intelligence algorithms. It takes into account a huge mass of data concerning real estate parameters, price history, the neighborhood, etc. The effectiveness of sales (or lack thereof), constantly calibrates the published offers. It is as if the system does not only understand and analyze the constantly changing data, but also makes decisions and proposes solutions to problems on the basis of its own conclusions.
Another level of AI development is the recently highly successful “Deep Learning” (DL). It is a category of methods that use neural networks, which is another genius mathematical invention (older than the concept of artificial intelligence itself), thanks to which we can talk about self-improvement, verification and processes which lead to autonomous (not supervised by man) inference, even from previously unsystematized data.

Autonomous cars and drones, Amazon GO stores, Google search results – all of these solutions are based on models of Deep Neural Networks in order to provide us with more life comfort, speed up taking decisions, and even compose songs for us.
Image recognition
For over thirty years people tried to teach machines to recognize objects in photographs by building mathematical models of faces, cars, trees, landscapes, suns, birds and etc. However, those attempts did not produce satisfactory results. For a time. When so many companies started to collect huge volumes of data, the need to use them emerged. Thanks to solutions such as CUDA (parallel processing) in NVIDIA graphics cards, data engineers have gained an unprecedented opportunity to quickly process terabytes of data on affordable hardware.
Due to its saturation with silicon and a huge increase in computing power, artificial intelligence began to learn how to recognize objects in images using patterns that serve as input data for neural networks. The more reference photos, the more effectively neural networks learn to identify them correctly. This means that less time and human work involving tedious and – let’s be honest – also boring, correction of results is needed to train the network to recognize Citroen C4s, Lenovo laptops, smiles, badgers or peonies correctly.
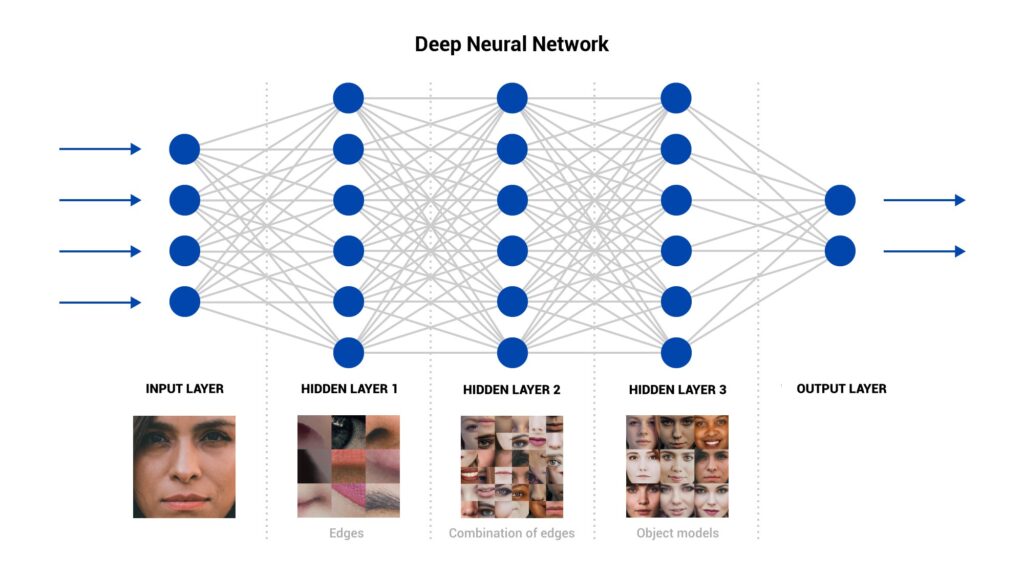
The recognition is optimized on the basis of a test set of images. As a result, we receive a well-trained neural network for use in a variety of applications, such as virtual fitting rooms, the portrait mode in smartphones or more lyrical contexts, such as Deep Dream Generator. However, applications of image recognition technology do not end there – it can also be used with success in business and commerce.
What could go wrong?
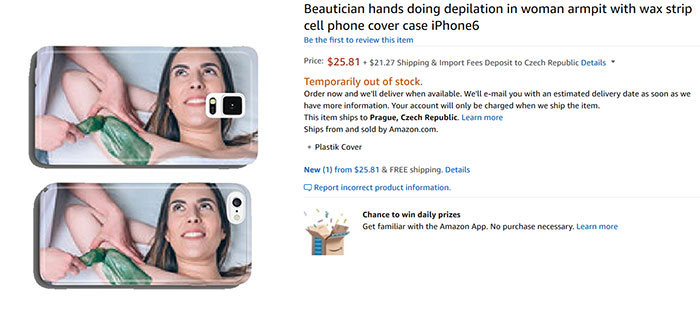
The potential of well-implemented artificial intelligence seems infinite. A technology company that does not research and develop solutions based on artificial intelligence will not find room for itself on the market of the future.
Product recognition – the key to knowledge
eLeader has specialized in solutions that help manufacturers and distributors make the best use of commercial mobile sales teams. Data from the field are reports from the first line of the front line of the fight for a store shelf. Acquiring these takes time, requires fuel consumption and a transfer of knowledge. It is therefore desirable that there are as many data as possible and that they are useful. The more visits, the more opportunities for contracts and increased sales, and the better relationships with stores. The more extensive and reliable the data, the better the sales strategy. However, the tedious counting of products, analysis of shelf shares and checking for shortages are prone to errors resulting from disturbances in perception, fatigue and often routine. So how to obtain more reliable data while reducing the time of the visit? How to obtain information quickly and immediately know what to do with it?
Since 2014 our clients have been using eLeader Shelf Recognition AI, the first solution on the market that enables analysis of a store shelf on the basis of its photographs and feeding data to the SFA system. More on the solution from eLeader can be found here.
Meet Ala
In order for us to be able to recognize images effectively – in this case, products from the shop shelf – we need templates. The more there are of them, the better. These templates are passed on to Ala (the name of eLeader neural network). In this way the stage of training eLeader Shelf Recognition AI is implemented. The artificial intelligence trained in this way is now fully suited to work with sales representatives, and photos taken on the fly during shop visits can be used for additional calibration.
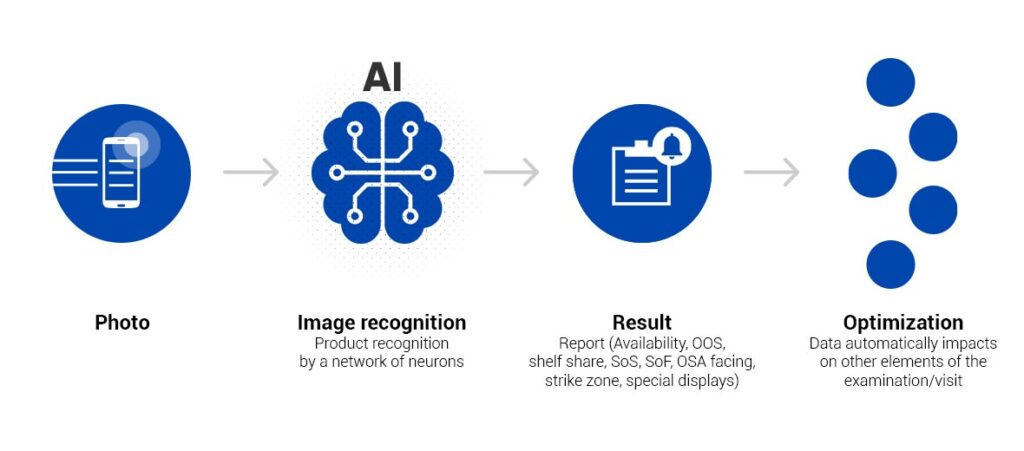
The process of implementation and release of the Shelf Recognition AI environment with Ala ready to work takes very little time. Once the project is up and running, mobile employees receive the results of automated product analysis during the same visit, which means that they can compare facts with the standard, e.g. Perfect Store, and immediately implement corrective measures in order to improve their and the organization’s KPIs. The information obtained and analyzed by this technology can be easily transformed into knowledge that forms the basis for strategic thinking on the market. For example, analyzed data from products help to create a company “store ranking”, according to which future visits are planned. As a result, mobile workers are more likely to appear where sales are highest (according to the RAO model, i.e. Retail Activities Optimization).
Read also:
- Sales visit scenario – what elements does the ideal one include?
- Surveys in the work of a sales representative – 14 examples that will help you understand your sales
- Planning and optimizing sales representatives’ routes, step by step
- Monitoring the Work of Sales Representatives
Soon in your fridge?
Intelligent product recognition is not only the future of business processes. The largest manufacturers of household appliances (featuring in the portfolio of eLeader Samsung, Whirlpool or LG) are improving devices that are now able not only to inform us about the upcoming expiry date (easy-peasy), but they are also able to communicate with each other by forwarding from the oven to the refrigerator, for example what we ate for lunch, to better adjust the dishwasher parameters or inform us that Kubuś carrot juice from Maspex is about to finish (i.e. identify its type and quantity, and then order it right through the fridge panel to be delivered to your door – that is easy, too). The Internet of things described here will soon transform a saying known from the world of politics or advertising into: you open the fridge and… AI is already there!
Regardless of how much we are going to use artificial intelligence and what an important place it will take in our lives or business, we must remember that the intelligence of the system depends primarily on the intelligence, knowledge and experience of AI engineers, creators and network trainers (Sorry, Ala), thanks to whom we can develop and expand eLeader Shelf Recognition AI and make the world a more enjoyable place.